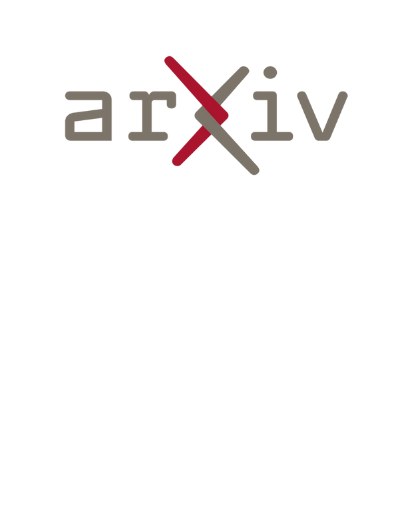
Human Wellbeing and Machine Learning
ARXIV
Ekaterina Oparina, Caspar Kaiser, Niccolò Gentile, Alexandre Tkatchenko, Andrew E. Clark, Jan-Emmanuel De Neve, Conchita D’Ambrosio
There is a vast literature on the determinants of subjective wellbeing. International organisations and statistical offices are now collecting such survey data at scale. However, standard regression models explain surprisingly little of the variation in wellbeing, limiting our ability to predict it. In response, we here assess the potential of Machine Learning (ML) to help us better understand wellbeing. We analyse wellbeing data on over a million respondents from Germany, the UK, and the United States. In terms of predictive power, our ML approaches do perform better than traditional models. Although the size of the improvement is small in absolute terms, it turns out to be substantial when compared to that of key variables like health. We moreover find that drastically expanding the set of explanatory variables doubles the predictive power of both OLS and the ML approaches on unseen data. The variables identified as important by our ML algorithms – i.e. material conditions, health, and meaningful social relations – are similar to those that have already been identified in the literature. In that sense, our data-driven ML results validate the findings from conventional approaches.